Handling datasets with multiple modalities¶
[1]:
%load_ext autotime
%config InlineBackend.figure_format = 'retina'
import scarf
scarf.__version__
[1]:
'0.8.5'
time: 991 ms (started: 2021-08-22 18:47:00 +00:00)
1) Fetch and convert data¶
For this tutorial we will use CITE-Seq data from 10x genomics. This dataset contains two modalities: gene expression and surface protein abundance. Throughout this tutorial we will refer to gene expression modality as RNA
and surface protein as ADT
. We start by downloading the data and converting it into Zarr format:
[2]:
scarf.fetch_dataset('tenx_8K_pbmc_citeseq', save_path='scarf_datasets')
INFO: Download started...
INFO: Download finished! File saved here: /home/docs/checkouts/readthedocs.org/user_builds/scarf/checkouts/0.8.5/docs/source/vignettes/scarf_datasets/tenx_8K_pbmc_citeseq/data.h5
time: 10.2 s (started: 2021-08-22 18:47:01 +00:00)
2021-08-22 18:47:11 URL:https://storage.googleapis.com/cos-osf-prod-files-de-1/6d69deb9c1868e6441996e1593fce74e5a3222bdee834bc90e1b77581991eb1e?response-content-disposition=attachment%3B%20filename%3D%22data.h5%22%3B%20filename%2A%3DUTF-8%27%27data.h5&GoogleAccessId=files-de-1%40cos-osf-prod.iam.gserviceaccount.com&Expires=1629658088&Signature=mnBj0uV7BgnxR4BvxL4MTcRPr9FC2NyTP%2Ba0Hd2L%2Fm%2FGJFPMwgv5DN%2FAn2Yr0rmryuByiGRfB8%2F4riiTXPXXUyG8QM%2BGnuUsNSeD6qRRUAMeDnXOelHkcapjQ1aaBYGxvfHOC4AdYQzKv50PxgvZ%2FYOCIW1Nqsu3sEap74Tnk%2BExOcf5A8JgiRZYS3sWHS72U%2F6R3xAwZTnbcDBshDIDuw5sG5ugNDOVQVfaj5hotfkrmJCdmXAiyl9bDSMKOX8J7m4gRh8OloUpmZHvBq1XpwmH5w577xhJqf3x8s6y8ByHIv8nJbv0c5cX4CSZM3%2F7KDXlYsGg1sQbjm4vE%2FmQMQ%3D%3D [21788278/21788278] -> "/home/docs/checkouts/readthedocs.org/user_builds/scarf/checkouts/0.8.5/docs/source/vignettes/scarf_datasets/tenx_8K_pbmc_citeseq/data.h5" [1]
[3]:
reader = scarf.CrH5Reader('scarf_datasets/tenx_8K_pbmc_citeseq/data.h5', 'rna')
time: 30.1 ms (started: 2021-08-22 18:47:11 +00:00)
We can also quickly check the different kinds of assays present in the file and the number of features from each of them.
[4]:
reader.assayFeats
[4]:
RNA | assay2 | |
---|---|---|
type | Gene Expression | Antibody Capture |
start | 0 | 33538 |
end | 33538 | 33555 |
nFeatures | 33538 | 17 |
time: 6.49 ms (started: 2021-08-22 18:47:11 +00:00)
The nFeatures column shows the number of features present in each assay. CrH5Reader
will automatically pull this information from H5 file and rename the ‘Gene Expression’ assay to RNA. Here it also found another assay: ‘Antibody Capture’ and named it to assay2. We will rename this to ADT.
[5]:
reader.rename_assays({'assay2': 'ADT'})
reader.assayFeats
[5]:
RNA | ADT | |
---|---|---|
type | Gene Expression | Antibody Capture |
start | 0 | 33538 |
end | 33538 | 33555 |
nFeatures | 33538 | 17 |
time: 4.81 ms (started: 2021-08-22 18:47:11 +00:00)
Now the data is converted into Zarr format. Like single assay datasets, all the data is saved under one Zarr file.
[6]:
writer = scarf.CrToZarr(reader, zarr_fn='scarf_datasets/tenx_8K_pbmc_citeseq/data.zarr',
chunk_size=(2000, 1000))
writer.dump(batch_size=1000)
100%|██████████| 8/8 [00:04<00:00, 1.64it/s]
time: 5.01 s (started: 2021-08-22 18:47:11 +00:00)
2) Create a multimodal DataStore¶
The next step is to create a Scarf DataStore
object. This object will be the primary way to interact with the data and all its constituent assays. The first time a Zarr file is loaded, we need to set the default assay. Here we set the ‘RNA’ assay as the default assay. When a Zarr file is loaded, Scarf checks if some per-cell statistics have been calculated. If not, then nFeatures (number of features per cell) and nCounts (total sum of feature counts per cell) are calculated. Scarf
will also attempt to calculate the percent of mitochondrial and ribosomal content per cell.
[7]:
ds = scarf.DataStore('scarf_datasets/tenx_8K_pbmc_citeseq/data.zarr',
default_assay='RNA',
nthreads=4)
INFO: Setting assay ADT to assay type: ADTassay
INFO: (ADT) Computing nCells and dropOuts
[########################################] | 100% Completed | 0.1s
INFO: Setting assay RNA to assay type: RNAassay
INFO: (RNA) Computing nCells and dropOuts
[########################################] | 100% Completed | 1.0s
INFO: (ADT) Computing nCounts
[########################################] | 100% Completed | 0.1s
INFO: (ADT) Computing nFeatures
[########################################] | 100% Completed | 0.1s
INFO: (RNA) Computing nCounts
[########################################] | 100% Completed | 0.9s
WARNING: Minimum cell count (501) is lower than size factor multiplier (1000)
INFO: (RNA) Computing nFeatures
[########################################] | 100% Completed | 1.0s
INFO: Computing percentage of RNA_percentMito
[########################################] | 100% Completed | 0.7s
INFO: Computing percentage of RNA_percentRibo
[########################################] | 100% Completed | 0.7s
time: 5.15 s (started: 2021-08-22 18:47:16 +00:00)
We can print out the DataStore object to get an overview of all the assays stored.
[8]:
ds
[8]:
DataStore has 7865 (7865) cells with 2 assays: ADT RNA
Cell metadata:
'I', 'ids', 'names', 'ADT_nCounts', 'ADT_nFeatures',
'RNA_nCounts', 'RNA_nFeatures', 'RNA_percentMito', 'RNA_percentRibo'
ADT assay has 17 (17) features and following metadata:
'I', 'ids', 'names', 'dropOuts', 'nCells',
RNA assay has 13832 (33538) features and following metadata:
'I', 'ids', 'names', 'dropOuts', 'nCells',
time: 5.14 ms (started: 2021-08-22 18:47:21 +00:00)
Feature attribute tables for each of the assays can be accessed like this:
[9]:
ds.RNA.feats.head()
[9]:
I | ids | names | dropOuts | nCells | |
---|---|---|---|---|---|
0 | False | ENSG00000243485 | MIR1302-2HG | 7865 | 0 |
1 | False | ENSG00000237613 | FAM138A | 7865 | 0 |
2 | False | ENSG00000186092 | OR4F5 | 7865 | 0 |
3 | False | ENSG00000238009 | AL627309.1 | 7853 | 12 |
4 | False | ENSG00000239945 | AL627309.3 | 7865 | 0 |
time: 14.8 ms (started: 2021-08-22 18:47:21 +00:00)
[10]:
ds.ADT.feats.head()
[10]:
I | ids | names | dropOuts | nCells | |
---|---|---|---|---|---|
0 | True | CD3 | CD3_TotalSeqB | 1 | 7864 |
1 | True | CD4 | CD4_TotalSeqB | 1 | 7864 |
2 | True | CD8a | CD8a_TotalSeqB | 2 | 7863 |
3 | True | CD14 | CD14_TotalSeqB | 1 | 7864 |
4 | True | CD15 | CD15_TotalSeqB | 1 | 7864 |
time: 13.9 ms (started: 2021-08-22 18:47:21 +00:00)
Cell filtering is performed based on the default assay. Here we use the auto_filter_cells
method of the DataStore
to filter low quality cells.
[11]:
ds.auto_filter_cells()
INFO: 154 cells flagged for filtering out using attribute RNA_nCounts
INFO: 326 cells flagged for filtering out using attribute RNA_nFeatures
INFO: 119 cells flagged for filtering out using attribute RNA_percentMito
INFO: 21 cells flagged for filtering out using attribute RNA_percentRibo
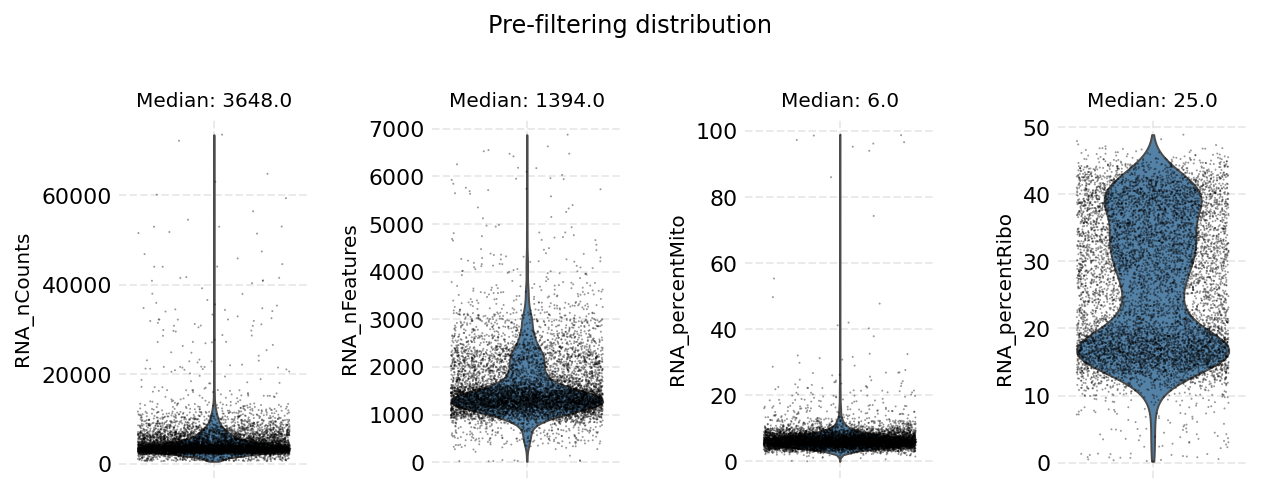
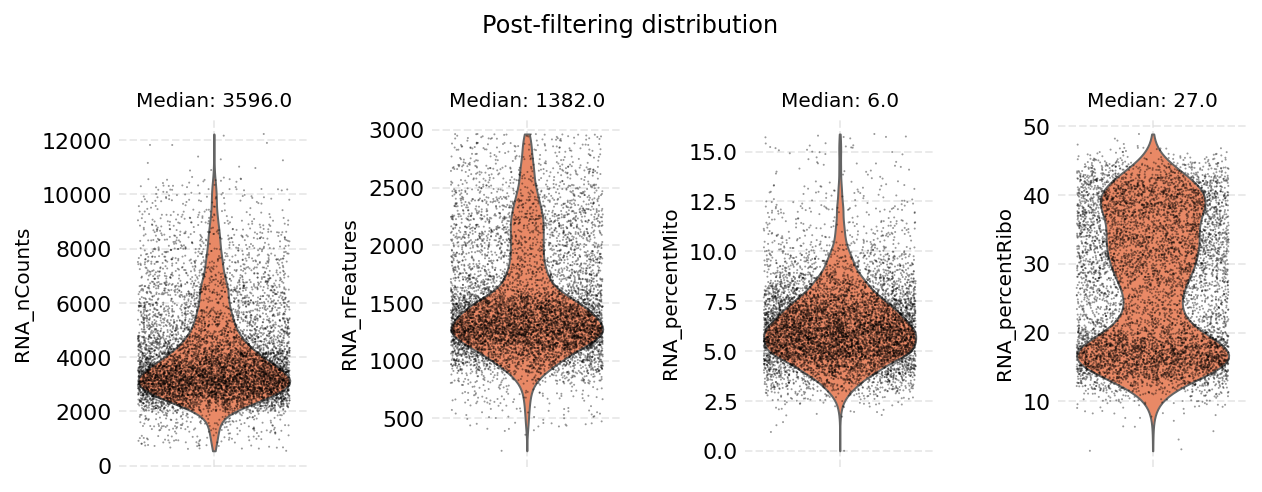
time: 2.21 s (started: 2021-08-22 18:47:21 +00:00)
3) Process gene expression modality¶
Now we process the RNA assay to perform feature selection, create KNN graph, run UMAP reduction and clustering. These steps are same as shown in the basic workflow for scRNA-Seq data.
[12]:
ds.mark_hvgs(min_cells=20, top_n=500, min_mean=-3, max_mean=2, max_var=6)
ds.make_graph(feat_key='hvgs', k=11, dims=15, n_centroids=100)
ds.run_umap(fit_n_epochs=250, spread=5, min_dist=1, parallel=True)
ds.run_leiden_clustering(resolution=1)
INFO: (RNA) Computing nCells
[########################################] | 100% Completed | 1.8s
INFO: (RNA) Computing normed_tot
[########################################] | 100% Completed | 1.7s
INFO: (RNA) Computing sigmas
[########################################] | 100% Completed | 2.0s
INFO: 497 genes marked as HVGs
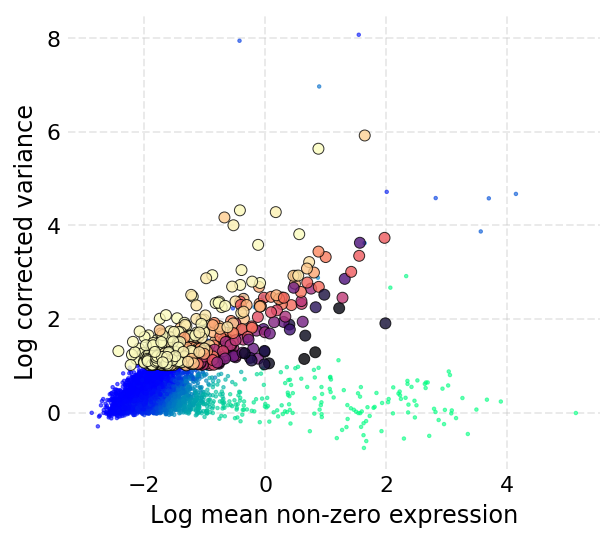
INFO: No value provided for parameter `log_transform`. Will use default value: True
INFO: No value provided for parameter `renormalize_subset`. Will use default value: True
INFO: No value provided for parameter `pca_cell_key`. Will use default value: I
INFO: Using PCA for dimension reduction
INFO: No value provided for parameter `ann_metric`. Will use default value: l2
INFO: No value provided for parameter `ann_efc`. Will use default value: min(100, max(k * 3, 50))
INFO: No value provided for parameter `ann_ef`. Will use default value: min(100, max(k * 3, 50))
INFO: No value provided for parameter `ann_m`. Will use default value: 48
INFO: No value provided for parameter `rand_state`. Will use default value: 4466
INFO: No value provided for parameter `local_connectivity`. Will use default value: 1.0
INFO: No value provided for parameter `bandwidth`. Will use default value: 1.5
INFO: Normalizing with feature subset
[########################################] | 100% Completed | 0.8s
Writing data to normed__I__hvgs/data: 100%|██████████| 4/4 [00:01<00:00, 3.24it/s]
INFO: Calculating mean of norm. data
[######## ] | 22% Completed | 0.1s
[########################################] | 100% Completed | 0.2s
INFO: Calculating std. dev. of norm. data
[########################################] | 100% Completed | 0.2s
Fitting PCA: 100%|██████████| 4/4 [00:01<00:00, 2.93it/s]
Fitting ANN: 100%|██████████| 4/4 [00:00<00:00, 6.80it/s]
Fitting kmeans: 100%|██████████| 4/4 [00:00<00:00, 5.33it/s]
Estimating seed partitions: 100%|██████████| 4/4 [00:00<00:00, 7.23it/s]
INFO: Saving loadings to RNA/normed__I__hvgs/reduction__pca__15__I
INFO: Saving ANN index to RNA/normed__I__hvgs/reduction__pca__15__I/ann__l2__50__50__48__4466
INFO: Saving kmeans clusters to RNA/normed__I__hvgs/reduction__pca__15__I/kmeans__100__4466
Saving KNN graph: 100%|██████████| 4/4 [00:00<00:00, 6.67it/s]
INFO: ANN recall: 99.81%
Smoothening KNN distances: 100%|██████████| 1/1 [00:02<00:00, 2.33s/it]
/home/docs/checkouts/readthedocs.org/user_builds/scarf/envs/0.8.5/lib/python3.8/site-packages/umap/umap_.py:1330: RuntimeWarning: divide by zero encountered in power
return 1.0 / (1.0 + a * x ** (2 * b))
completed 0 / 250 epochs
completed 25 / 250 epochs
completed 50 / 250 epochs
completed 75 / 250 epochs
completed 100 / 250 epochs
completed 125 / 250 epochs
completed 150 / 250 epochs
completed 175 / 250 epochs
completed 200 / 250 epochs
completed 225 / 250 epochs
completed 0 / 100 epochs
completed 10 / 100 epochs
completed 20 / 100 epochs
completed 30 / 100 epochs
completed 40 / 100 epochs
completed 50 / 100 epochs
completed 60 / 100 epochs
completed 70 / 100 epochs
completed 80 / 100 epochs
completed 90 / 100 epochs
time: 32.5 s (started: 2021-08-22 18:47:23 +00:00)
[13]:
ds.plot_layout(layout_key='RNA_UMAP', color_by='RNA_leiden_cluster')
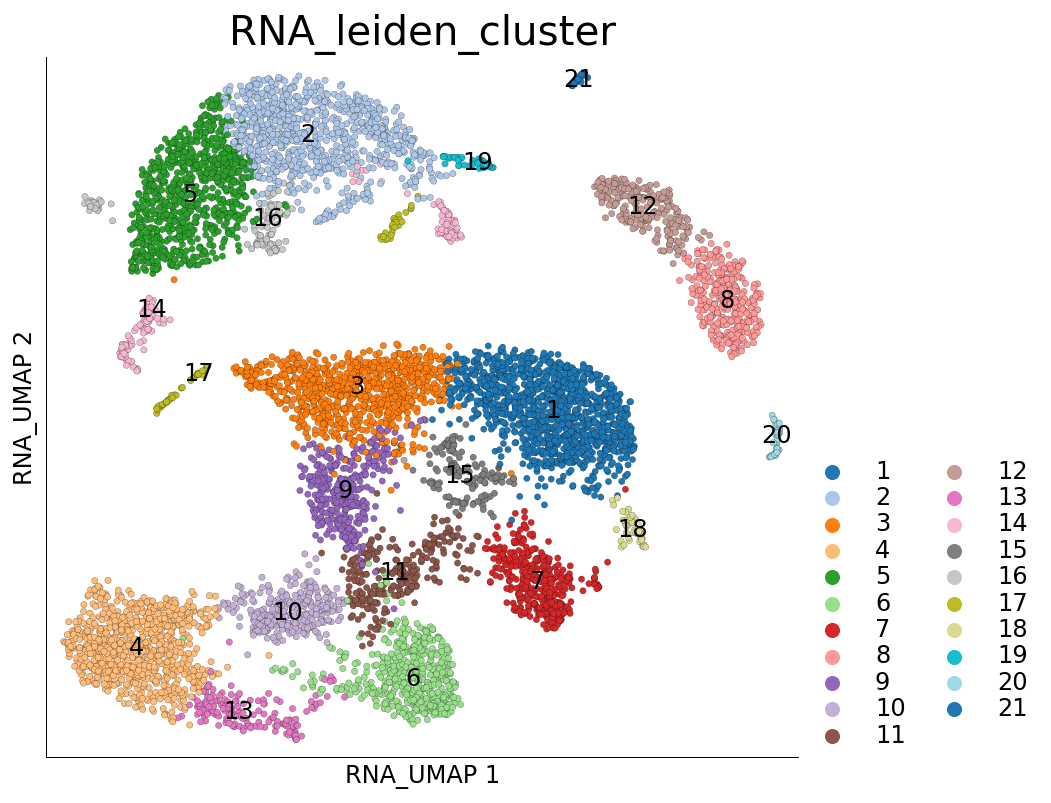
time: 777 ms (started: 2021-08-22 18:47:56 +00:00)
4) Process protein surface abundance modality¶
We will now perform similar steps as RNA for the ADT data. Since ADT panels are often custom designed, we will not perform any feature selection step. This particular data contains some control antibodies which we should filter out before downstream analysis.
[14]:
ds.ADT.feats.head(n=ds.ADT.feats.N)
[14]:
I | ids | names | dropOuts | nCells | |
---|---|---|---|---|---|
0 | True | CD3 | CD3_TotalSeqB | 1 | 7864 |
1 | True | CD4 | CD4_TotalSeqB | 1 | 7864 |
2 | True | CD8a | CD8a_TotalSeqB | 2 | 7863 |
3 | True | CD14 | CD14_TotalSeqB | 1 | 7864 |
4 | True | CD15 | CD15_TotalSeqB | 1 | 7864 |
5 | True | CD16 | CD16_TotalSeqB | 1 | 7864 |
6 | True | CD56 | CD56_TotalSeqB | 1 | 7864 |
7 | True | CD19 | CD19_TotalSeqB | 163 | 7702 |
8 | True | CD25 | CD25_TotalSeqB | 4 | 7861 |
9 | True | CD45RA | CD45RA_TotalSeqB | 1 | 7864 |
10 | True | CD45RO | CD45RO_TotalSeqB | 1 | 7864 |
11 | True | PD-1 | PD-1_TotalSeqB | 2 | 7863 |
12 | True | TIGIT | TIGIT_TotalSeqB | 16 | 7849 |
13 | True | CD127 | CD127_TotalSeqB | 3 | 7862 |
14 | True | IgG2a | IgG2a_control_TotalSeqB | 26 | 7839 |
15 | True | IgG1 | IgG1_control_TotalSeqB | 9 | 7856 |
16 | True | IgG2b | IgG2b_control_TotalSeqB | 226 | 7639 |
time: 14.4 ms (started: 2021-08-22 18:47:57 +00:00)
We can manually filter out the control antibodies by updating I to be False for those features. To do so we first extract the names of all the ADT features like below:
[15]:
adt_names = ds.ADT.feats.to_pandas_dataframe(['names'])['names']
adt_names
[15]:
0 CD3_TotalSeqB
1 CD4_TotalSeqB
2 CD8a_TotalSeqB
3 CD14_TotalSeqB
4 CD15_TotalSeqB
5 CD16_TotalSeqB
6 CD56_TotalSeqB
7 CD19_TotalSeqB
8 CD25_TotalSeqB
9 CD45RA_TotalSeqB
10 CD45RO_TotalSeqB
11 PD-1_TotalSeqB
12 TIGIT_TotalSeqB
13 CD127_TotalSeqB
14 IgG2a_control_TotalSeqB
15 IgG1_control_TotalSeqB
16 IgG2b_control_TotalSeqB
Name: names, dtype: object
time: 8.64 ms (started: 2021-08-22 18:47:57 +00:00)
The ADT features with ‘control’ in name are designated as control antibodies. You can have your own selection criteria here. The aim here is to create a boolean array that has True
value for features to be removed.
[16]:
is_control = adt_names.str.contains('control').values
is_control
[16]:
array([False, False, False, False, False, False, False, False, False,
False, False, False, False, False, True, True, True])
time: 2.48 ms (started: 2021-08-22 18:47:57 +00:00)
Now we update I
to remove the control features. update_key
method takes a boolean array and disables the features that have False
value. So we invert the above created array (using ~
) before providing it to update_key
. The second parameter for update_key
denotes which feature table boolean column to modify, I
in this case.
[17]:
ds.ADT.feats.update_key(~is_control, 'I')
ds.ADT.feats.head(n=ds.ADT.feats.N)
[17]:
I | ids | names | dropOuts | nCells | |
---|---|---|---|---|---|
0 | True | CD3 | CD3_TotalSeqB | 1 | 7864 |
1 | True | CD4 | CD4_TotalSeqB | 1 | 7864 |
2 | True | CD8a | CD8a_TotalSeqB | 2 | 7863 |
3 | True | CD14 | CD14_TotalSeqB | 1 | 7864 |
4 | True | CD15 | CD15_TotalSeqB | 1 | 7864 |
5 | True | CD16 | CD16_TotalSeqB | 1 | 7864 |
6 | True | CD56 | CD56_TotalSeqB | 1 | 7864 |
7 | True | CD19 | CD19_TotalSeqB | 163 | 7702 |
8 | True | CD25 | CD25_TotalSeqB | 4 | 7861 |
9 | True | CD45RA | CD45RA_TotalSeqB | 1 | 7864 |
10 | True | CD45RO | CD45RO_TotalSeqB | 1 | 7864 |
11 | True | PD-1 | PD-1_TotalSeqB | 2 | 7863 |
12 | True | TIGIT | TIGIT_TotalSeqB | 16 | 7849 |
13 | True | CD127 | CD127_TotalSeqB | 3 | 7862 |
14 | False | IgG2a | IgG2a_control_TotalSeqB | 26 | 7839 |
15 | False | IgG1 | IgG1_control_TotalSeqB | 9 | 7856 |
16 | False | IgG2b | IgG2b_control_TotalSeqB | 226 | 7639 |
time: 14.1 ms (started: 2021-08-22 18:47:57 +00:00)
Assays named ADT are automatically created as objects of the ADTassay
class, which uses CLR (centred log ratio) normalization as the default normalization method.
[18]:
print (ds.ADT)
print (ds.ADT.normMethod.__name__)
ADTassay ADT with 14(17) features
norm_clr
time: 2.14 ms (started: 2021-08-22 18:47:57 +00:00)
Now we are ready to create a KNN graph of cells using only ADT data. Here we will use all the features (except those that were filtered out) and that is why we use I
as value for feat_key
. It is important to note the value for from_assay
parameter which has now been set to ADT
. If no value is provided for from_assay
then it is automatically set to the default assay.
[19]:
ds.make_graph(from_assay='ADT', feat_key='I', k=11, dims=11, n_centroids=100)
INFO: No value provided for parameter `log_transform`. Will use default value: True
INFO: No value provided for parameter `renormalize_subset`. Will use default value: True
INFO: No value provided for parameter `pca_cell_key`. Will use default value: I
INFO: Using PCA for dimension reduction
INFO: No value provided for parameter `ann_metric`. Will use default value: l2
INFO: No value provided for parameter `ann_efc`. Will use default value: min(100, max(k * 3, 50))
INFO: No value provided for parameter `ann_ef`. Will use default value: min(100, max(k * 3, 50))
INFO: No value provided for parameter `ann_m`. Will use default value: 48
INFO: No value provided for parameter `rand_state`. Will use default value: 4466
INFO: No value provided for parameter `local_connectivity`. Will use default value: 1.0
INFO: No value provided for parameter `bandwidth`. Will use default value: 1.5
Writing data to normed__I__I/data: 100%|██████████| 4/4 [00:00<00:00, 9.20it/s]
INFO: Calculating mean of norm. data
[################# ] | 44% Completed | 0.1s
[########################################] | 100% Completed | 0.2s
INFO: Calculating std. dev. of norm. data
[########################################] | 100% Completed | 0.2s
Fitting PCA: 100%|██████████| 4/4 [00:00<00:00, 13.69it/s]
Fitting ANN: 100%|██████████| 4/4 [00:00<00:00, 6.87it/s]
Fitting kmeans: 100%|██████████| 4/4 [00:00<00:00, 5.70it/s]
Estimating seed partitions: 100%|██████████| 4/4 [00:00<00:00, 7.65it/s]
INFO: Saving loadings to ADT/normed__I__I/reduction__pca__11__I
INFO: Saving ANN index to ADT/normed__I__I/reduction__pca__11__I/ann__l2__50__50__48__4466
INFO: Saving kmeans clusters to ADT/normed__I__I/reduction__pca__11__I/kmeans__100__4466
Saving KNN graph: 100%|██████████| 4/4 [00:00<00:00, 8.81it/s]
INFO: ANN recall: 99.97%
Smoothening KNN distances: 100%|██████████| 1/1 [00:00<00:00, 34.47it/s]
time: 3.6 s (started: 2021-08-22 18:47:57 +00:00)
UMAP and clustering can be run on ADT assay by simply setting from_assay
parameter value to ‘ADT’:
[20]:
ds.run_umap(from_assay='ADT', fit_n_epochs=250, spread=5, min_dist=1, parallel=True)
ds.run_leiden_clustering(from_assay='ADT', resolution=1)
/home/docs/checkouts/readthedocs.org/user_builds/scarf/envs/0.8.5/lib/python3.8/site-packages/umap/umap_.py:1330: RuntimeWarning: divide by zero encountered in power
return 1.0 / (1.0 + a * x ** (2 * b))
completed 0 / 250 epochs
completed 25 / 250 epochs
completed 50 / 250 epochs
completed 75 / 250 epochs
completed 100 / 250 epochs
completed 125 / 250 epochs
completed 150 / 250 epochs
completed 175 / 250 epochs
completed 200 / 250 epochs
completed 225 / 250 epochs
completed 0 / 100 epochs
completed 10 / 100 epochs
completed 20 / 100 epochs
completed 30 / 100 epochs
completed 40 / 100 epochs
completed 50 / 100 epochs
completed 60 / 100 epochs
completed 70 / 100 epochs
completed 80 / 100 epochs
completed 90 / 100 epochs
time: 14.6 s (started: 2021-08-22 18:48:00 +00:00)
If we now check the cell attribute table, we will find the UMAP coordinates and clusters calculated using ADT
assay:
[21]:
ds.cells.head()
[21]:
I | ids | names | ADT_UMAP1 | ADT_UMAP2 | ADT_leiden_cluster | ADT_nCounts | ADT_nFeatures | RNA_UMAP1 | RNA_UMAP2 | RNA_leiden_cluster | RNA_nCounts | RNA_nFeatures | RNA_percentMito | RNA_percentRibo | |
---|---|---|---|---|---|---|---|---|---|---|---|---|---|---|---|
0 | True | AAACCCAAGATTGTGA-1 | AAACCCAAGATTGTGA-1 | -22.835373 | 15.652700 | 16 | 981.0 | 17.0 | -25.220272 | 23.941408 | 5 | 6160.0 | 2194.0 | 8.668831 | 15.259740 |
1 | True | AAACCCACATCGGTTA-1 | AAACCCACATCGGTTA-1 | -10.856400 | 21.918344 | 15 | 1475.0 | 17.0 | -22.625509 | 20.475191 | 5 | 6713.0 | 2093.0 | 6.316103 | 19.037688 |
2 | True | AAACCCAGTACCGCGT-1 | AAACCCAGTACCGCGT-1 | -13.277214 | 22.179325 | 7 | 7149.0 | 17.0 | -14.885433 | 22.384569 | 2 | 3637.0 | 1518.0 | 8.056090 | 16.002200 |
3 | True | AAACCCAGTATCGAAA-1 | AAACCCAGTATCGAAA-1 | 24.064547 | 19.403200 | 2 | 6831.0 | 17.0 | -29.751768 | -19.231924 | 4 | 1244.0 | 737.0 | 9.003215 | 18.729904 |
4 | True | AAACCCAGTCGTCATA-1 | AAACCCAGTCGTCATA-1 | 24.413895 | 15.854909 | 2 | 6839.0 | 17.0 | -25.344509 | -29.261696 | 4 | 2611.0 | 1240.0 | 6.204519 | 16.353887 |
time: 28.5 ms (started: 2021-08-22 18:48:15 +00:00)
Visualizing the UMAP and clustering calcualted using ADT
only:
[22]:
ds.plot_layout(layout_key='ADT_UMAP', color_by='ADT_leiden_cluster')
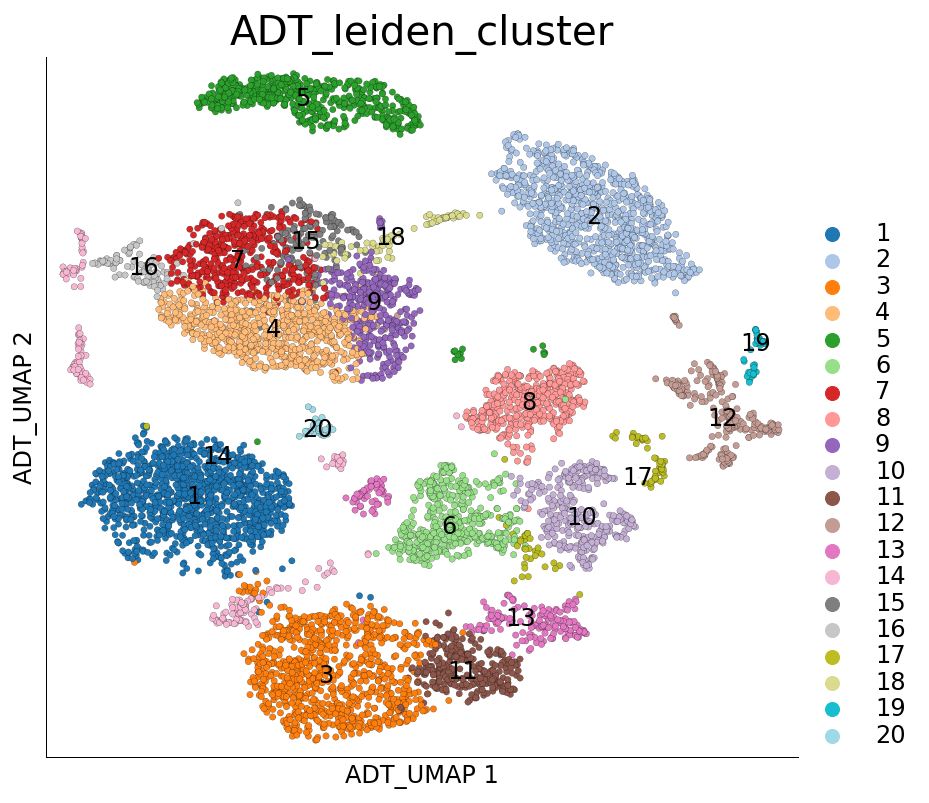
time: 734 ms (started: 2021-08-22 18:48:15 +00:00)
5) Cross modality comparison¶
It is generally of interest to see how different modalities corroborate each other.
[23]:
# UMAP on RNA and coloured with clusters calculated on ADT
ds.plot_layout(layout_key='RNA_UMAP', color_by='ADT_leiden_cluster')
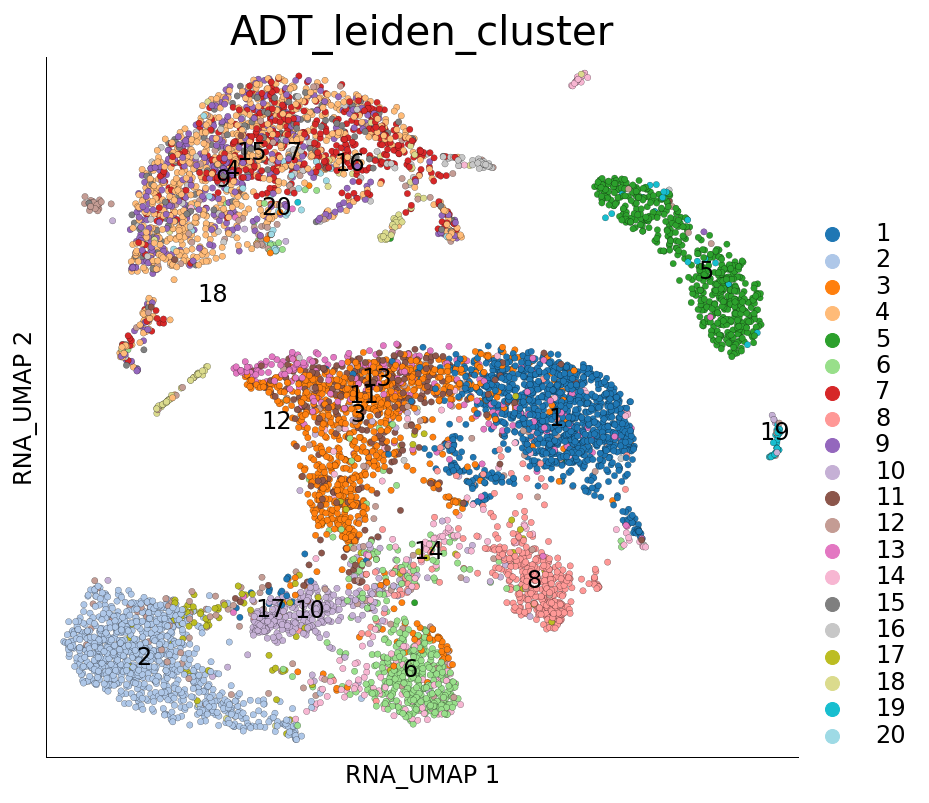
time: 732 ms (started: 2021-08-22 18:48:16 +00:00)
[24]:
# UMAP on ADT and coloured with clusters calculated on RNA
ds.plot_layout(layout_key='ADT_UMAP', color_by='RNA_leiden_cluster')
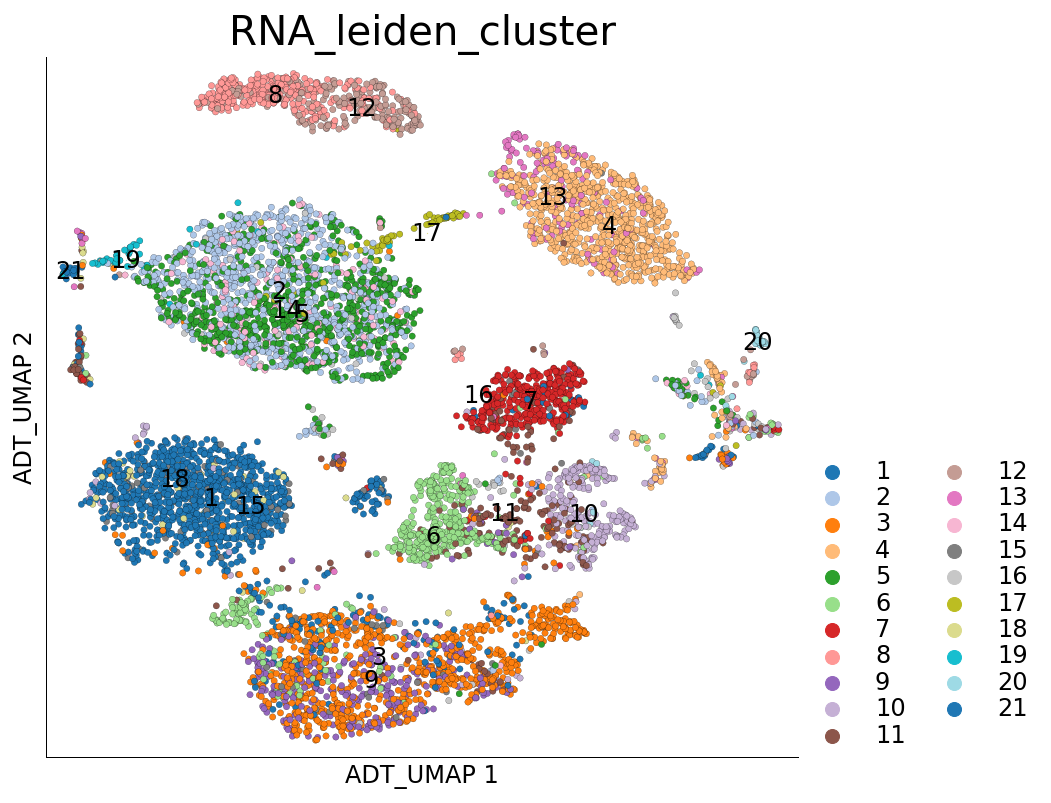
time: 761 ms (started: 2021-08-22 18:48:16 +00:00)
We can quantify the overlap of cells between RNA and ADT clusters. The following table has ADT clusters on columns and RNA clusters on rows. This table shows a cross tabulation of cells across the clustering from the two modalities.
[25]:
import pandas as pd
df = pd.crosstab(ds.cells.fetch('RNA_leiden_cluster'),
ds.cells.fetch('ADT_leiden_cluster'))
df
[25]:
col_0 | 1 | 2 | 3 | 4 | 5 | 6 | 7 | 8 | 9 | 10 | 11 | 12 | 13 | 14 | 15 | 16 | 17 | 18 | 19 | 20 |
---|---|---|---|---|---|---|---|---|---|---|---|---|---|---|---|---|---|---|---|---|
row_0 | ||||||||||||||||||||
1 | 928 | 0 | 83 | 0 | 1 | 0 | 0 | 15 | 0 | 1 | 18 | 17 | 67 | 17 | 0 | 1 | 2 | 0 | 0 | 0 |
2 | 0 | 0 | 1 | 290 | 0 | 3 | 296 | 0 | 111 | 1 | 3 | 23 | 0 | 0 | 76 | 35 | 0 | 11 | 1 | 11 |
3 | 17 | 0 | 428 | 3 | 0 | 4 | 0 | 1 | 0 | 9 | 175 | 21 | 134 | 8 | 0 | 1 | 4 | 1 | 0 | 0 |
4 | 0 | 735 | 0 | 0 | 0 | 0 | 0 | 0 | 0 | 3 | 0 | 35 | 0 | 0 | 0 | 0 | 31 | 0 | 0 | 0 |
5 | 0 | 0 | 0 | 349 | 0 | 3 | 83 | 0 | 192 | 0 | 2 | 23 | 1 | 1 | 60 | 22 | 0 | 4 | 0 | 7 |
6 | 0 | 2 | 47 | 0 | 0 | 330 | 0 | 2 | 0 | 7 | 0 | 14 | 0 | 74 | 0 | 0 | 9 | 0 | 0 | 0 |
7 | 0 | 0 | 0 | 0 | 0 | 5 | 0 | 335 | 0 | 4 | 0 | 4 | 1 | 7 | 0 | 0 | 7 | 0 | 0 | 0 |
8 | 0 | 0 | 0 | 0 | 312 | 0 | 0 | 0 | 0 | 0 | 0 | 1 | 1 | 0 | 0 | 0 | 0 | 0 | 7 | 0 |
9 | 2 | 0 | 235 | 0 | 1 | 11 | 0 | 1 | 0 | 2 | 44 | 11 | 1 | 5 | 0 | 0 | 3 | 0 | 0 | 0 |
10 | 16 | 1 | 7 | 0 | 0 | 3 | 0 | 3 | 0 | 220 | 8 | 17 | 2 | 1 | 0 | 0 | 17 | 0 | 0 | 0 |
11 | 1 | 1 | 2 | 0 | 1 | 61 | 0 | 43 | 0 | 62 | 15 | 12 | 1 | 42 | 0 | 0 | 8 | 0 | 0 | 0 |
12 | 0 | 0 | 0 | 0 | 208 | 0 | 0 | 0 | 1 | 0 | 1 | 3 | 0 | 0 | 0 | 2 | 0 | 0 | 11 | 0 |
13 | 0 | 134 | 0 | 0 | 0 | 2 | 0 | 2 | 0 | 0 | 0 | 5 | 0 | 10 | 0 | 1 | 4 | 3 | 0 | 0 |
14 | 0 | 0 | 0 | 58 | 0 | 1 | 34 | 0 | 29 | 0 | 1 | 9 | 0 | 0 | 13 | 1 | 0 | 2 | 0 | 2 |
15 | 81 | 1 | 27 | 0 | 0 | 0 | 0 | 9 | 0 | 1 | 7 | 3 | 6 | 3 | 0 | 0 | 1 | 0 | 0 | 0 |
16 | 0 | 1 | 4 | 20 | 0 | 11 | 5 | 0 | 7 | 3 | 1 | 36 | 2 | 0 | 1 | 0 | 0 | 0 | 0 | 10 |
17 | 0 | 0 | 0 | 4 | 1 | 0 | 3 | 0 | 0 | 0 | 0 | 5 | 0 | 0 | 0 | 0 | 0 | 59 | 0 | 0 |
18 | 31 | 0 | 1 | 0 | 0 | 2 | 0 | 0 | 0 | 0 | 1 | 0 | 3 | 10 | 0 | 1 | 0 | 0 | 0 | 0 |
19 | 0 | 0 | 0 | 1 | 0 | 0 | 3 | 0 | 0 | 0 | 0 | 2 | 0 | 2 | 1 | 32 | 0 | 1 | 0 | 0 |
20 | 0 | 0 | 0 | 0 | 0 | 0 | 0 | 0 | 0 | 5 | 0 | 4 | 0 | 0 | 0 | 0 | 0 | 0 | 30 | 0 |
21 | 0 | 0 | 0 | 0 | 0 | 0 | 0 | 0 | 0 | 0 | 0 | 0 | 0 | 21 | 0 | 0 | 0 | 1 | 0 | 0 |
time: 28.5 ms (started: 2021-08-22 18:48:17 +00:00)
There are possibly many interesting strategies to analyze this further. One simple way to summarize the above table can be quantify the transcriptomics ‘purity’ of ADT clusters:
[26]:
(100 * df.max()/df.sum()).sort_values(ascending=False)
[26]:
col_0
1 86.245353
2 84.000000
8 81.508516
6 75.688073
18 71.951220
7 69.811321
10 69.182390
11 63.405797
19 61.224490
13 61.187215
5 59.541985
9 56.470588
3 51.257485
15 50.331126
4 48.137931
14 36.815920
20 36.666667
16 36.458333
17 36.046512
12 14.693878
dtype: float64
time: 7.62 ms (started: 2021-08-22 18:48:17 +00:00)
Individual ADT expression can be visualized in both UMAPs easily.
[27]:
ds.plot_layout(from_assay='ADT', layout_key='ADT_UMAP', color_by='CD16_TotalSeqB')
ds.plot_layout(from_assay='ADT', layout_key='RNA_UMAP', color_by='CD16_TotalSeqB')
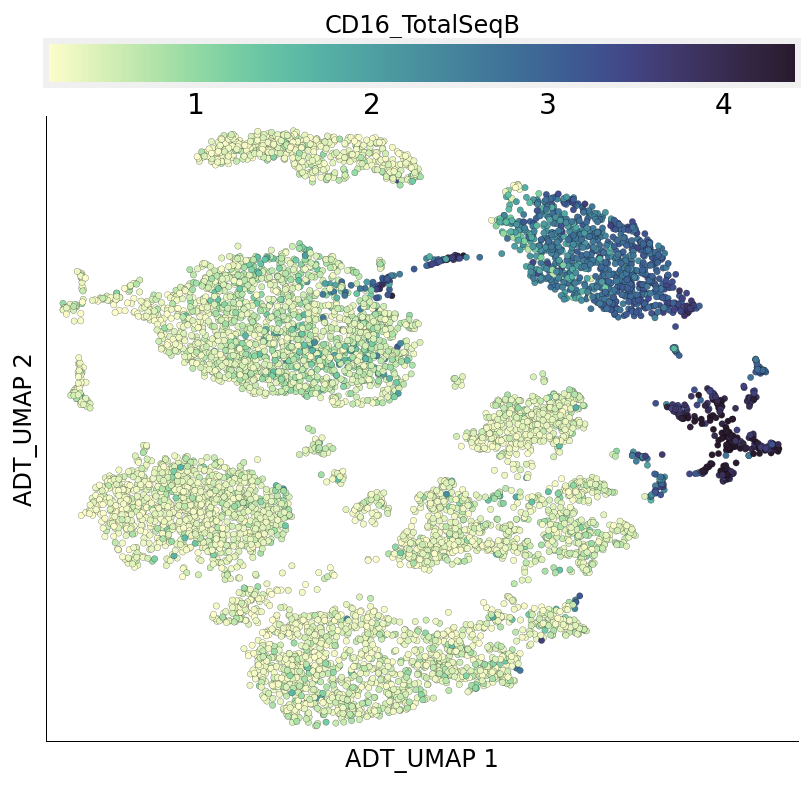
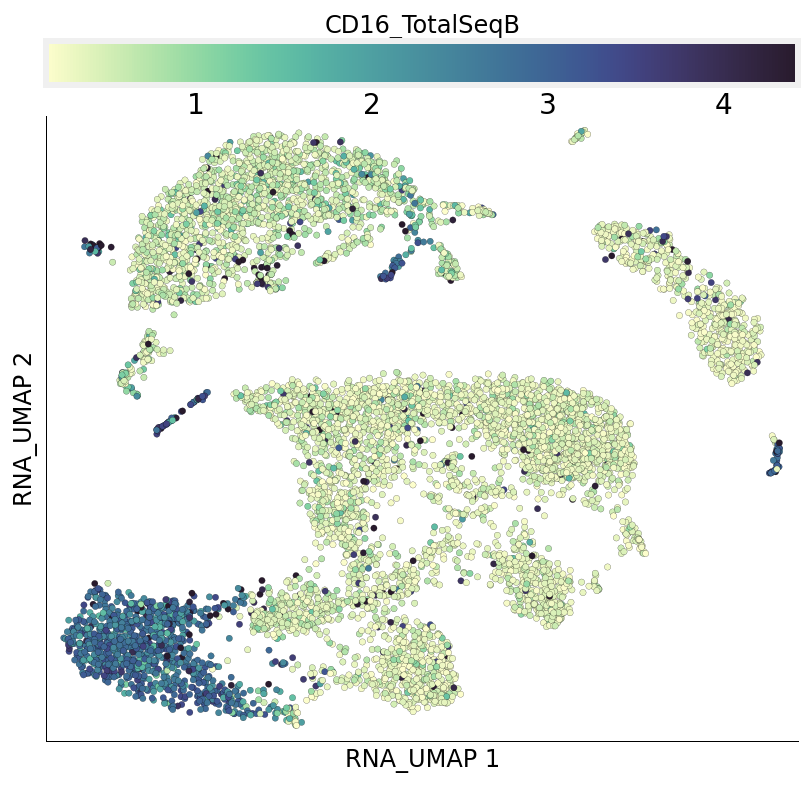
time: 1.93 s (started: 2021-08-22 18:48:17 +00:00)
We can also query gene expression and visualize it on both RNA and ADT UMAPs. Here we query gene FCGR3A which codes for CD16:
[28]:
ds.plot_layout(from_assay='RNA', layout_key='RNA_UMAP', color_by='FCGR3A')
ds.plot_layout(from_assay='RNA', layout_key='ADT_UMAP', color_by='FCGR3A')
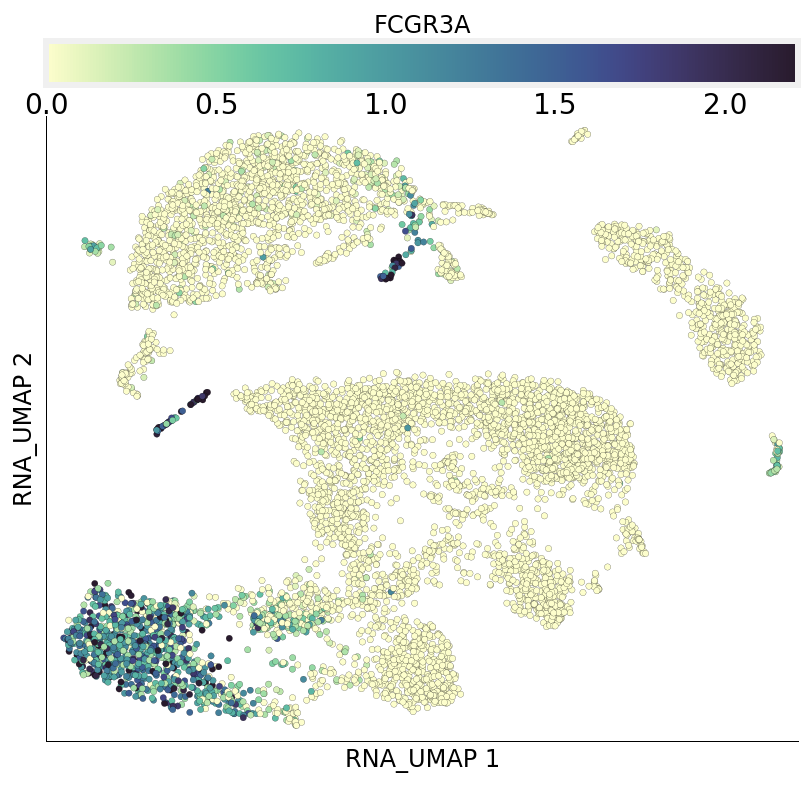
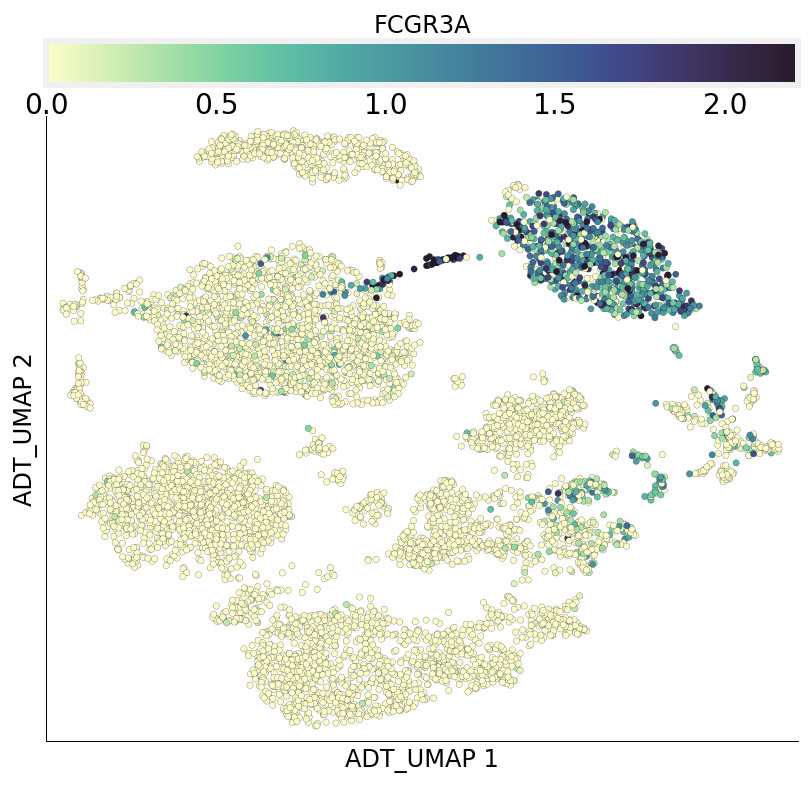
time: 2.11 s (started: 2021-08-22 18:48:19 +00:00)
That is all for this vignette.