Workflow for scATAC-Seq data¶
%load_ext autotime
import scarf
scarf.__version__
'0.16.3'
time: 1.01 s (started: 2021-08-22 17:24:45 +00:00)
1) Fetch and convert data¶
scarf.fetch_dataset('tenx_10K_pbmc_atacseq', save_path='scarf_datasets')
reader = scarf.CrH5Reader('scarf_datasets/tenx_10K_pbmc_atacseq/data.h5', 'atac')
reader.assayFeats
INFO: Download finished! File saved here: /home/docs/checkouts/readthedocs.org/user_builds/scarf/checkouts/0.16.3/docs/source/vignettes/scarf_datasets/tenx_10K_pbmc_atacseq/data.h5
ATAC | |
---|---|
type | Peaks |
start | 0 |
end | 90686 |
nFeatures | 90686 |
time: 9.49 s (started: 2021-08-22 17:24:46 +00:00)
writer = scarf.CrToZarr(reader, zarr_fn=f'scarf_datasets/tenx_10K_pbmc_atacseq/data.zarr', chunk_size=(1000, 2000))
writer.dump(batch_size=1000)
time: 21.8 s (started: 2021-08-22 17:24:56 +00:00)
2) Create DataStore and filter cells¶
ds = scarf.DataStore('scarf_datasets/tenx_10K_pbmc_atacseq/data.zarr', nthreads=4)
time: 8.61 s (started: 2021-08-22 17:25:18 +00:00)
ds.auto_filter_cells()
INFO: 296 cells flagged for filtering out using attribute ATAC_nCounts
INFO: 260 cells flagged for filtering out using attribute ATAC_nFeatures
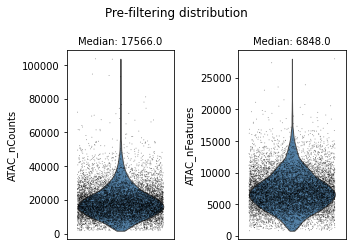
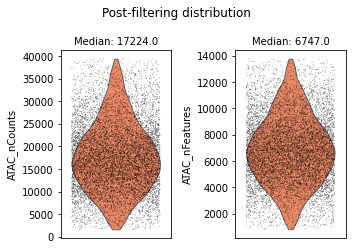
time: 3.83 s (started: 2021-08-22 17:25:26 +00:00)
3) Feature selection¶
For scATAC-Seq data, the features are ranked by their TF-IDF normalized values, summed across all cells. The top n features are marked as prevalent_peaks
and are used for downstream steps.
ds.mark_prevalent_peaks(top_n=20000)
time: 9.21 s (started: 2021-08-22 17:25:30 +00:00)
4) KNN graph creation¶
For scATAC-Seq datasets, Scarf uses TF-IDF normalization. The normalization is automatically performed during the graph building step. The selected features, marked as prevalent_peaks
in feature metadata, are used for graph creation. For the dimension reduction step, LSI (latent semantic indexing) is used rather than PCA. The rest of the steps are same as for scRNA-Seq data.
ds.make_graph(feat_key='prevalent_peaks', k=11, dims=21, n_centroids=1000)
/home/docs/checkouts/readthedocs.org/user_builds/scarf/envs/0.16.3/lib/python3.8/site-packages/gensim/similarities/__init__.py:15: UserWarning: The gensim.similarities.levenshtein submodule is disabled, because the optional Levenshtein package <https://pypi.org/project/python-Levenshtein/> is unavailable. Install Levenhstein (e.g. `pip install python-Levenshtein`) to suppress this warning.
warnings.warn(msg)
INFO: ANN recall: 99.99%
time: 2min 29s (started: 2021-08-22 17:25:39 +00:00)
5) UMAP reduction and clustering¶
Non-linear dimension reduction using UMAP and tSNE are performed in the same way as for scRNA-Seq data. Similarly the clustering step is also performed in the same way as for scRNA-Seq data.
ds.run_umap(n_epochs=250, min_dist=0.5, parallel=True)
completed 0 / 250 epochs
completed 25 / 250 epochs
completed 50 / 250 epochs
completed 75 / 250 epochs
completed 100 / 250 epochs
completed 125 / 250 epochs
completed 150 / 250 epochs
completed 175 / 250 epochs
completed 200 / 250 epochs
completed 225 / 250 epochs
time: 8.86 s (started: 2021-08-22 17:28:08 +00:00)
ds.run_leiden_clustering(resolution=1)
time: 222 ms (started: 2021-08-22 17:28:17 +00:00)
ds.plot_layout(layout_key='ATAC_UMAP', color_by='ATAC_leiden_cluster')
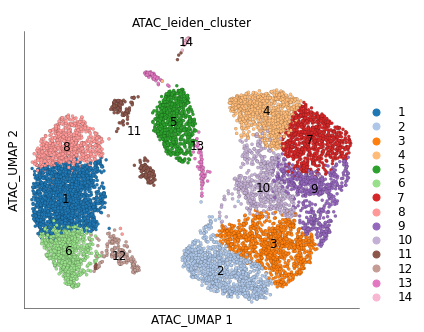
time: 556 ms (started: 2021-08-22 17:28:18 +00:00)
6) Calculating gene scores¶
This feature is coming soon..
ds.ATAC.feats.head()
I | ids | names | I__prevalent_peaks | dropOuts | nCells | stats_I_prevalence | |
---|---|---|---|---|---|---|---|
0 | True | chr1:565163-565491 | chr1:565163-565491 | False | 9619 | 49 | 0.123722 |
1 | True | chr1:569190-569620 | chr1:569190-569620 | False | 9545 | 123 | 0.299672 |
2 | True | chr1:713551-714783 | chr1:713551-714783 | True | 6403 | 3265 | 2.214712 |
3 | True | chr1:752418-753020 | chr1:752418-753020 | False | 9102 | 566 | 0.557693 |
4 | True | chr1:762249-763345 | chr1:762249-763345 | True | 7433 | 2235 | 1.673136 |
time: 23.8 ms (started: 2021-08-22 17:28:18 +00:00)
That is all for this vignette.